Sustainable AI computing for IoT
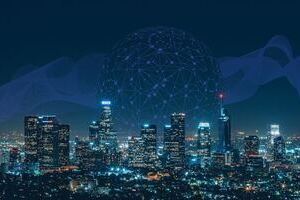
AI and Computing can, if not properly planned, be extremely heavy and wasteful of CPU resources. To put it in perspective, the computing power required for AI landmarks, such as recognising images or defeating humans at Go, increased 300,000-fold from 2012 to 2018.
Other studies indicate that training an AI language-processing system produced 1,400 pounds (635.03 kg) of emission, which is roughly the same amount needed to fly one person on a roundtrip between New York and San Francisco, says Mattias Bergstrom, lead product architect & chairman at Internet of Everything Corp.
Given the exponential growth in energy consumption to train AI, for full sustainability thinking, even the use of what algorithms and methods should be evaluated. And in IoT devices deployment, for example, using floating windows for pattern mining in data streams is many times more energy-efficient than processing all the data.
This is especially efficient in IoT as the data generated that needs to be refined into information is not constant, but bursting. For example, a traffic intersection is at most times empty, but has short periods of intense rushes, constantly using full computing is automatically being wasteful. So if we want to solve problems for mankind with smart solutions, those solutions need to be sustainable.
Data to information refinement
In the introduction, we have presented the immense energy-consumption AI computing, currently requires, but it is also true that AI is one of the drivers of IoT. As such, a balance has to be made between the AI processing data and its energy consumption to achieve the best results to solve humankind’s problems via smart solutions.
There is no point in solving Cities’ traffic problems and reducing pollution, if the solution is not sustainable. That means that if the solution relies on third-party systems, or utilises computing that has unfixed pricing, smart solutions are destined to become burdens for the cities and other installations. To better understand the inefficiencies of third-party systems, e.g., cloud providers, let’s look at some facts:
- The average computer wastes nearly half the power it consumes.
- Servers lose approximately one-third of their power as heat.
- It’s predicted ICT’s global emissions by 2025 can rise to up to 3.5% surpassing aviation and shipping.
Taking this data into consideration, one of the solutions that is being presented is data to information (D2I) refinement. A principle that acts upon Information and Communication Technology’s (ICT) energy consumption, by clustering IoT devices to compute clusters. In this way, heat generation can be limited as computing is balanced over the devices, and as the devices in an IoT installation are spread out it is not generated in one room.
Using power-aware compute clusters, the computed load can be balanced so that none of the devices run hot. Creating a green and sustainable Cloud of Things, enabling resource-sharing clustered edge computing. Which is more than some simple data pre-processing, taking advantage of only a small portion of the computing that is possible on the edge. This infrastructure creates, what is known as Private Online Gardens with meaningful Edge Computing, which in turn allows for data to information refinement at a local level. Providing a system that doesn’t require to be constantly sending data to server centres, and thus, it isn’t bound to internet connectivity for it to function.
Do IoT devices need servers?
Well no, no they don’t. The benefit of IoT installations is that the Internet of Everything can have access to computing power at the installation. A process possible with the aforementioned clustering IoT devices to compute clusters, where data can be stored and processed on the devices directly (the so-called edge). A solution that allows for useful and refined Information to be available for services to access and use, reducing the networking traffic.
The result of this innovative technology reduces computing power used in data centres tremendously because it makes use of the computing resources of devices that are already in use. This greatly reduces the bandwidth and energy required by data centres. In addition, edge computing also provides the flexibility to operate independently of an Internet connection, enables fast real-time response rates, and cuts cloud costs.
Sustainable AI computing for smart home
In practice, the mentioned above works, for example, at a smart home’s thermostat’s IoT device which might produce 1000s of temperature data points per minute. However, minimal changes typically do not matter, and data updates aren’t necessary every millisecond, therefore, all this data in the cloud and accessible from anywhere isn’t necessary.
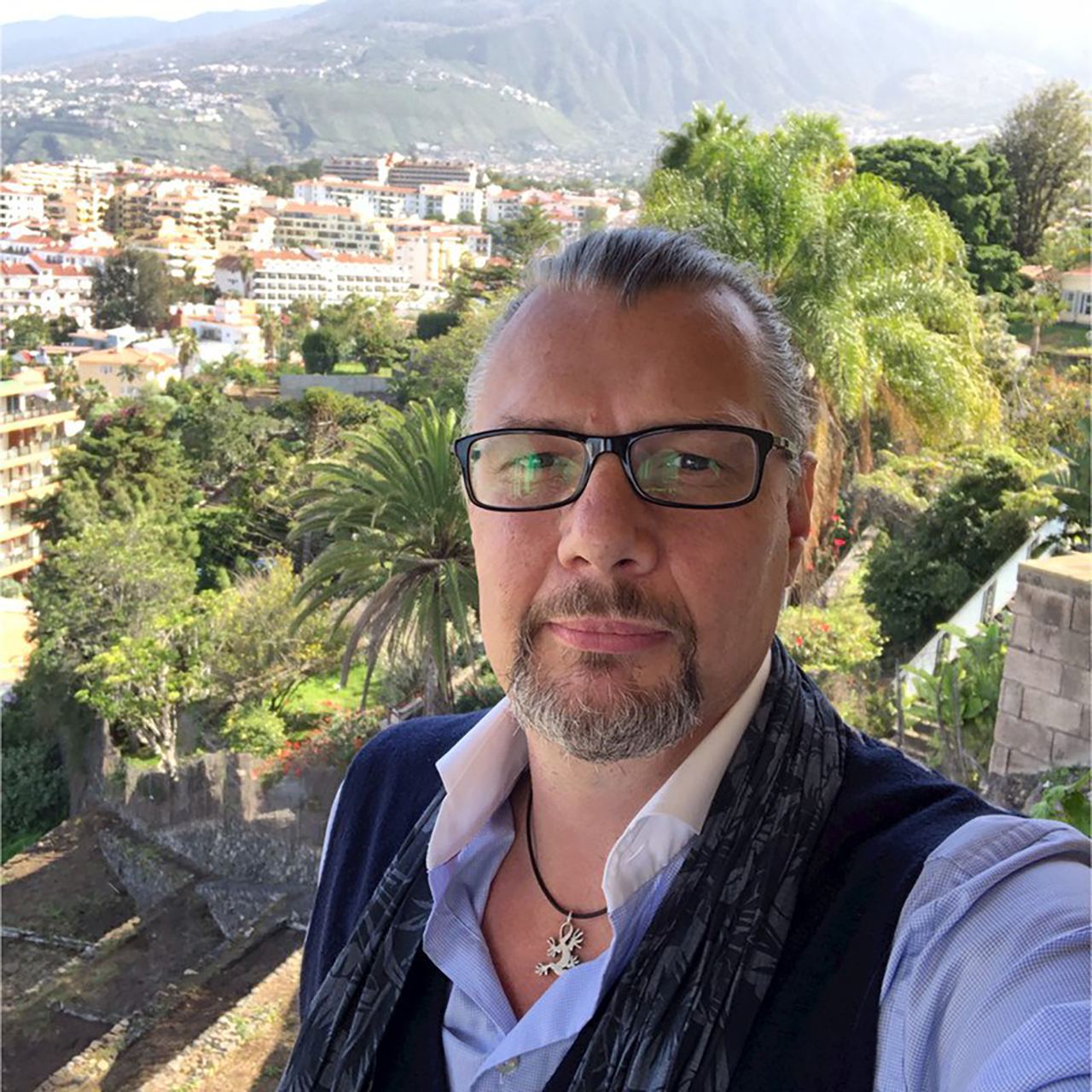
Mattias Bergstrom
With clustered edge computing, this data can stay in the cluster and will be used within the smart home as needed. Clustered edge computing enables the smart home to work fast, efficiently, and autonomously from a working internet connection. In addition, the smart homeowner can keep the private data to him/herself and is less vulnerable to cyberattacks.
Having this system operational for smart solutions, triggers a sustainable AI computing that provides D2I refinement, and works on the edge offering real-time information. In conclusion, we can reduce the unnecessary use of bandwidth and server capacity (which comes down to infrastructure, electricity, and physical space) while simultaneously taking advantage of smart device resources. Amplifying these benefits, with high performance on small devices and efficient data synchronisation makes clustered edge computing the most sustainable AI and computing solution for IoT.
The author is Mattias Bergstrom, lead product architect & chairman at Internet of Everything Corp
About the author
Mattias is a successful innovator, serial entrepreneur, and futurist focusing on business and technical strategies of distributed networks, AI, and computing-based solutions. He is a huge believer in the Internet, and technology overall, for good. Currently, he supports Governments and Fortune 500 with groundbreaking technology and strategic advice.
Comment on this article below or via Twitter @IoTGN