Data management and data analytics: A synergistic relationship
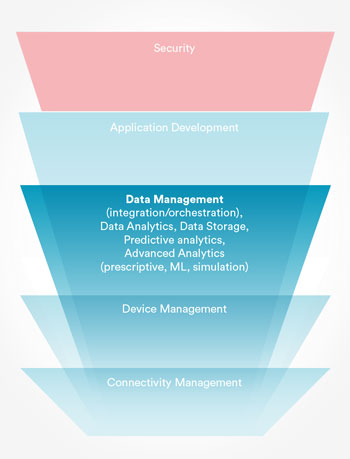
Connectivity management enables data calls to be made to devices. Devices generate data, which is the fuel that powers the IoT, but the value of that data depends on what you do with it. The first step comes from data management services, which increase operational efficiency by monitoring and maintaining the performance of remote devices and improving business processes by enabling seamless sharing of data across users and devices. Therefore it’s hard to overstate the importance of data management’s role in IoT solutions, although it hasn’t figured front and centre in recent years. Nevertheless, it should be seen as a mandatory first step in unlocking IoT’s potential.
As the deployment of IoT devices continues to rise, so does the volume of data that needs to be stored, processed and analysed. Cisco predicts that by 2020 IoT will be generating 600 Zettabytes annually (1 ZB=1 trillion GBs) of data and this figure is set to grow. So, where is it going to be stored, is it necessary to store every byte, and how is it going to be managed?
Until recently virtually all device data was sent to a central facility, a private or public cloud, where it was stored, processed, managed and analysed in order to obtain insightful information. However, this process takes time, not a lot of time, but it means that the information is historic: it is not “in the moment”.
Edge computing, which is a relatively recent development, reduces the volume of data that needs to be retained and transmitted to a central facility. It enables data to be processed and analysed locally in commodity servers or gateways that are de facto local clouds. This process generates real-time information, which enables fast decisions to be made at the local level.
Therefore, data management services and data analytics are now employed in both centralised and local clouds that are distributed around the network.
Data analytics’ deliverables
We can divide data analytics into long term analytics employed in a central facility, where it is used to discover hidden patterns, unknown correlations, market trends, customer preferences and other useful business information. And real time data analysis performed in a local facility, where it is being used to create preventative maintenance programs that detect potential issues before they occur and provide real-time information on manufacturing operations.
In addition combinations of long-term and real-time data analytics enable predictive models that are typically used to analyse current and historic data in order to better understand customers, products and partners and to identify potential risks and opportunities for a company.
Machine learning
Machine learning is the logical, next step on the path towards Artificial Intelligence (AI), but it should also be seen as an evolutionary development since it is basically an extension of regular predictive analytics. The applications employ algorithms that predict outcomes based on input data and the accuracy of the prediction improves over time as the result of new input data. This enables systems to learn automatically and improve from experience without being explicitly programmed.
Unlike AI, machine learning doesn’t have a hype curve. It’s a pragmatic technology that is being applied across the industrial landscape. Applications include facial recognition, video surveillance, traffic predictions, spam and malware filtering, healthcare, online customer support and so on. It’s a long list.
Machine learning is becoming a business-critical technology that is enabling decisions to be based on data-driven processes. Therefore, it is logical to assume that we will witness systems that combine machine learning with established data analytic solutions in order to realise AI functionality.
Conclusions
Data flows into companies from myriad sources, but what makes it a precious commodity depends on what you do with it. Data management is a mandatory first step in unlocking IoT’s potential, followed by data analytics, which provides insightful information on the way that businesses are performing. However, connectivity and device management are critical components and as IoT expands both in terms of reach and functionality post deployment performance is not something that can be taken for granted. Therefore when evaluating IoT platforms and selecting the optimum platform for your operation you need to consider vendor credentials in all three areas.