Data analytics is driving IoT’s future
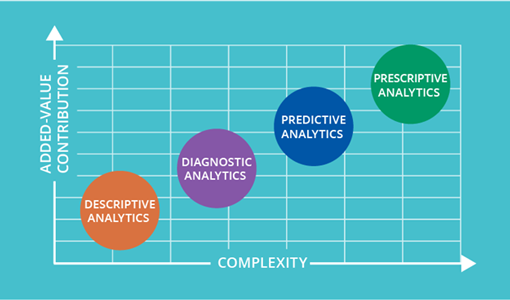
IoT analytics can be divided into two broad categories: device data analytics and network analytics. A further division can be made into descriptive, diagnostic, predictive and prescriptive analytics. These are generic terms that predate IoT, but they are just as relevant today. The early analysis of enterprise data, e.g. data generated by mainstream systems like CRM and ERP, was and still is done with a Business Intelligence platform.
Descriptive Analytics as the simplest form of data analytics: it provides information about something that happened. It does not investigate or establish cause and effect relationships. The objective is to present facts and figures to either inform or prepare data for further analysis. The information is often presented in pie charts, bar charts, line graphs and tables.
Diagnostic Analytics provides information about why that something happened. It establishes dependencies and identifies patterns therefore proving in-depth insights into a particular problem. At the same time it generates detailed information that companies can use for other purposes.
Predictive Analytics provides information on what is likely to happen. It employs the results of descriptive and diagnostic analytics in order to detect tendencies, clusters and exceptions, and to predict future trends. However the predictions are only estimates and they depend on the quality of the data quality and the stability of the situation that is being analysed.
Prescriptive Analytics provides information on what action can be taken prevent something happening. It can also be used to benefit from emerging business and marketing trends. Prescriptive analytics uses sophisticated tools and technologies like machine learning as well as business rules and algorithms.
IoT analytics
IoT device data maps to descriptive, diagnostic, predictive and prescriptive analytics. For example, descriptive flags the status of devices; diagnostic indicates which device has failed and why; predictive identifies which device looks likely to fail; and prescriptive would put the device into a suspended state until it was fixed. Therefore the value of these four models increases in line with the complexity of the task that is being analysed, as illustrated in the graphic. In a nutshell, it grows from hindsight to insight and on to foresight.
A similar exercise can be made for connectivity. It might start with an alert about the connection to a particular device being lost. Diagnosis could indicate that there was no coverage in that area. Predictive could indicate that eight devices were close to the coverage area and therefore there might be connectivity issues. And prescriptive might switch the connection to an alternative area.
Integrated analytics
In IoT solutions the functionality of these four models is enabled by processing and analysing device data using advanced analysis tools and the application of machine learning technology, which is an extension of the predictive analysis model. Preventative maintenance programs, for example, have become a core component of industrial IoT solutions.and data analytics is becoming an integrated component of IoT platforms. Moreover its importance will grow in line with the upcoming increase in data traffic, as evidenced by the development of IoT analytics platforms and managed analytics services hosted in public clouds.
Data analytics will gain momentum in the foreseeable future and it will be at the core of countless new technology solutions. The options created by machine learning and artificial intelligence are virtually endless and business intelligence dashboards, used in conjunction with enterprise-class data analytics tools, give management at all levels a better understanding what is going on inside the company.
Conclusions
Data is the fuel that powers the IoT, but its value comes from the ways that we analyse and use it to deliver tangible benefits, e.g. boost operational efficiency, cut costs and enable the development of new business models. Preventative maintenance programs were an early way and employing real-time data to deliver insightful information on manufacturing processes is a relatively recent development that industry now takes for granted.
Data is managed and analysed in central facilities, private and publish clouds, as well as local clouds that are distributed around the network. Central facilities deliver historic information generated by mainstream systems and device data. Local facilities deliver real-time information that allows management decisions to be taken locally, in the moment.
Data is the fuel that powers the IoT. Data analytics is driving its future.