Machine Learning: Making sure you can walk before you run
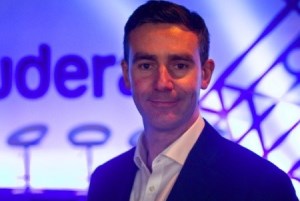
Stephen Line
There are few technological innovations that present businesses with new opportunities to the extent that machine learning (ML) does. A multitude of approaches, including deep learning image classifications and natural language processing are already delivering transformative benefits across industries.
From using data to reduce risk exposure in insurance, to deriving greater insight from customer information in the utilities industry, businesses that succeed in applying machine learning for business at scale will inevitably be at the forefront of positive change in their industry, says Stephen Line vice president of EMEA at Cloudera. However, while most business leaders have heard of ML, comparatively few genuinely understand how to implement a strategic and practical roadmap to apply it for business value.
For some, the language and terminology that surrounds ML is confusing enough to pose barriers to progress. For others, the difference between ML and artificial intelligence (AI) creates uncertainty due to the concerns about replacing people with machines. Some have even invested in and deployed ML technologies, but do not realise that is what they have.
Overcoming these challenges is essential for every IT and business leader. Not least because the rewards for using ML solutions have allowed many businesses that have invested to realise tangible returns on their investment. Harnessing the opportunity provided by ML is the way forward for IT executives who want to make a meaningful and transformative difference to their organisation.
Back to basics
Machine learning has been the tech buzzword for some time now, and the results can be seen in how businesses perceive the trend. A study of IT decision-makers from more than 15 different industries across four countries – the UK, France, Germany and Spain – found that 87% of companies have implemented ML technology or plan to do so by 2020. This makes machine learning the second highest current investment priority for businesses, just behind analytics but ahead of IoT, AI, and data science.
However, there seems to be a lack of clarity around what machine learning is and does for businesses, with many not understanding what ML’s impact could be for their business. In the aforementioned study of European businesses, only half understood ML use cases and how it works. Furthermore, fewer than a third have an established team of data scientists with contemporary ML skills, with half getting their ML know-how through IT news sites.
While understanding the importance of ML and adopting or intending to adopt ML solutions within an organisation is a great step forward, if the knowledge base and resources at the organisation are non-existent, then businesses will never achieve the ROI they want.
The barriers to machine learning success
Despite the fact that ML is essentially a process of data analysis to improve system performance, one of the most common barriers to adoption has its roots in the old sci-fi narrative of man versus machine. Put simply, among today’s IT leaders there remains widespread mistrust in the ability of technology to replace human decision making. Other key barriers to entry are lack of resources, lack of knowledge, costs, and cultural resistance.
Businesses are missing out on the benefits of ML for reasons that can easily be solved by outsourcing, drawing on external expertise, and growing their understanding of what ML is and what it does. Bringing in external expertise will help them to understand the delineation between AI and ML, and to understand where it can transform their busses – may that be operation efficiency, better customer service through data, or greater financial and resource efficiency.
The key to realising ROI is having a clear understanding of why you are looking to ML, what benefits it will realise and how it will transform the business. Importantly, this strategic approach will be the thing that overcomes the barriers. It is key for ML-ambitious IT leaders to improve how they demonstrate that an ML solution can tangibly and clearly solve a business problem or deliver a business benefit. For example, if there is a will to improve customer service, there is a case to say that ML can bring siloed data sets together for a more holistic customer view. If the need is operational efficiency, then demonstrating how ML can enhance the way people do their jobs rather than replacing them with a robot is the way to go.
Make sure to walk before you run
Machine learning presents a huge opportunity for businesses. With it they can better use the vast amounts of data points they collect, see it all in context, and use ML solutions to make better decisions on everything from customer service to security, while realising huge efficiency and profit gains. However, this is all being held back by a great many businesses suffering from below optimum levels of understanding about what ML is and does.
Too many see it as aligned to AI – taking jobs away instead of using intelligent processes to help people do their jobs better. Others struggle to know who should be driving ML adoption, so business cases are hard to build and ROI hard to come by. However, by taking an ML strategy back to basics, making sure everyone understands the processes, thinking strategically and using outside help and resources, every business can work smarter, do things faster, and make previously impossible tasks routine.
The author of the blog is Stephen Line, vice president EMEA, Cloudera
Comment on this article below or via Twitter @IoTGN