Why all your data matters for IoT business impact – Part Two
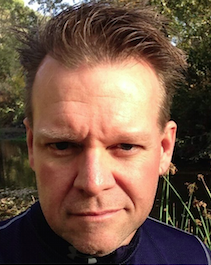
Patrick McFadin, DataStax
Data from the heating system should not be seen on its own, writes Patrick McFadin, a Cassandra Evangelist at DataStax. Overall energy consumption data can and should be viewed alongside air conditioning and water usage information, while businesses with large energy use for specific purposes like refrigeration can break out this information too. In this example, putting these sets of data alongside each other can help manage the facility more efficiently but also look to spot other less obvious patterns in the data.
In this case, energy may be consumed at a higher rate than is usual for the facility. The normal response might be to turn down the power automatically, but is this the right course of action? Is the energy cost out of line with other similar locations or facilities that the company might be running? Or is this an isolated case due to local weather or another outside factor?
It’s only by combining these data sets for analysis over time, and then using that data for real time actions, that IoT strategies can have the required impact. This involves using the results of time-series data analytics alongside new data that is being created, so that operational and analytic data can be processed side by side.
Drawing data together
Alongside this, there are other ways to collate data and use it for the future. One alternative approach to modelling this data in a more effective way is to use a graph database system. This describes a specific form of database that is particularly suited to showing the relationships that exist across data sets.
Originally developed and used for large sets of data that did not fit in traditional table or relational schemas, the use case for graph databases has developed to model data sets where the complex connections between items are important for generating insight into relationships, such as with social media analytics data. This approach can be applied to the data created by IoT devices as well.
The aim here is to create insight into the relationships that are being created between users IoT services and everything in between. These complex relationships can inform future activity by the customer and by the company providing the service. For example, looking at patterns around usage over time can help show where there are opportunities to affect user behaviour in positive ways compared to default settings. By comparing patterns and relationships using graph, it’s possible to find new relationships and insights compared to what is possible using other database management systems on their own.
However, the key challenge for IoT is to bring all these different systems together. Alongside graph data, the time-series data itself has great potential value for analytics, while the analysis of actions should take place in as near real-time as possible in order to create more value for the business. This approach means bringing together different database management systems into one overall approach to working with data.
This consolidation and tight integration of database technologies, analytics and management information will provide more insight to IoT services providers. In turn, this insight can be turned into new services for customers, leading to more potential revenues over time. Similarly, enterprises can look at their data to find more opportunities for efficiency or cost reductions in their service offerings. By bringing these different forms of data together, IoT can then start to deliver on its promise.